AI-BLACK BOX PHENOMENON
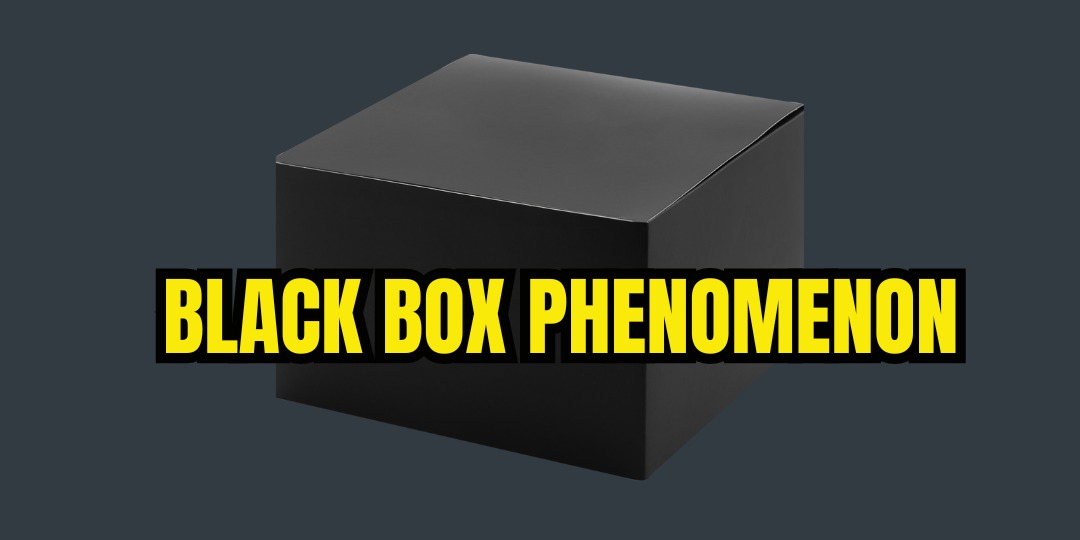
Sai Meera .D – Principal Associate & Patent Agent, Intellectual Property
Although we are looking at adopting and adapting to AI both in our daily lives and making maximum use in professional lives. AI’s path to mainstream adoption is riddled with hurdles in development, deployment, and use. Overcoming these challenges is key to unlocking its true potential. Though promising, AI faces roadblocks across its lifecycle. To truly integrate it into our world, we must tackle issues from creation to application. AI’s practical successes like Tesla’s self-driving cars and fraud detection systems shine a spotlight on its potential. However, acknowledging the challenges involved paves the way for responsible and sustainable development.1
One of the critical risks in current Artificial Intelligence models is the Black Box Phenomenon.
The events that made us contemplate on the reliability of AI and its risks.
The first serious accident involving a self-driving car in Australia occurred in March this year. A pedestrian suffered life-threatening injuries when hit by a Tesla Model 3, which the driver claims was in “autopilot” mode. In the US, the highway safety regulator is investigating a series of accidents where Teslas on autopilot crashed into first-responder vehicles with flashing lights during traffic stops. The decision-making processes of “self-driving” cars are often opaque and unpredictable (even to their manufacturers), so it can be hard to determine who should be held accountable for incidents such as these.3
The AI deep learning models where there is Lack of Transparency is termed as Black Box Phenomenon.3 Since AI drew its inspiration from the human brain, it is presumed that the early learning in children pattern is followed or rather programmed to be followed by AI.
The basic theory is that the brain is a trend-finding machine. Brain reads the examples and coalesces into patterns and derives its conclusions. Doing this is easy. Explaining how we do this is essentially impossible. “It’s one of those weird things that you know, but you don’t know how you know it or where you learned it,” says Associate Professor of Electrical and Computer Engineering Samir Rawashdeh, who specializes in artificial intelligence.2
Deep learning, one of the most ubiquitous modern forms of artificial intelligence, works much the same way, in no small part because it was inspired by this theory of human intelligence. Deep learning algorithms are trained much the same way we teach children. The system is fed with correct examples of something that one want it to be able to recognize, and before long, its own trend-finding inclinations will have worked out a “neural network” for categorizing things it’s never experienced before. As we have never figured out the potential of human brain in learning, similarly we have no idea of how a deep learning system comes to its conclusions. It is presumed that deep learning has “lost track” of the inputs that informed its decision making a long time ago, or to put it as it was never keeping track.
This inability for us, the users to see how deep learning systems make their decisions is known as the “Black box problem,” and it’s a real concern. First, this quality makes it difficult to fix deep learning systems when they produce unwanted outcomes. The black box phenomenon is one where the outcome cannot be traced back to answering why the AI chose to present that particular outcome.
Deep learning systems are now regularly used to make judgements about humans in contexts ranging from medical treatments, to who should get approved for a loan, to which applicants should get a job interview. In each of these areas, it’s been demonstrated that AI systems can reflect unwanted biases from our human world. Needless to say, a deep learning system that can deny you a loan or screen you out of the first round of job interviews but can’t explain why, is one most people would have a hard time judging as “fair.”
Two Machine-Learning Algorithms Widely Used in AI and the Black Box Problem
One possible reason AI may be a black box to humans is that it relies on machine-learning algorithms that internalize data in ways that are not easily audited or understood by humans. First, a lack of transparency may arise from the complexity of the algorithm’s structure, such as with a deep neural network, which consists of thousands of artificial neurons working together in a diffuse way to solve a problem. This reason for AI being a black box is referred to as “complexity.” Second, the lack of transparency may arise because the AI is using a machine-learning algorithm that relies on geometric relationships that humans cannot visualize, such as with support vector machines. This reason for AI being a black box is referred to as “dimensionality.”
1. Deep Neural Networks and Complexity The deep neural network is based on a mathematical model called the artificial neuron. While originally based on a simplistic model of the neurons in human and animal brains, the artificial neuron is not meant to be a computer-based simulation of a biological neuron. Instead, the goal of the artificial neuron is to achieve the same ability to learn from experience as with the biological neuron. Deep Neural Networks, where several layers of interconnected neurons are used to progressively find patterns in data or to make logical or relational connections between data points. Deep networks of artificial neurons have been used to recognize images, even detecting cancer at levels of accuracy exceeding that of experienced doctors.
Support Vector Machines and Dimensionality Some machine-learning algorithms are opaque to human beings because they arrive at decisions by looking at many variables at once and finding geometric patterns among those variables that humans cannot visualize.
Generally, the Black Box Problem can be defined as an inability to fully understand an AI’s decision-making process and the inability to predict the AI’s decisions or outputs. However, whether an AI’s lack of transparency will have implications for intent and causation tests depends on the extent of this lack of transparency. A complete lack of transparency will in most cases result in the complete failure of intent and causation tests to function, but some transparency may allow these tests to continue functioning, albeit to a limited extent.
Types of Black Boxes
Strong Black Boxes: Strong black boxes are AI with decision making processes that are entirely opaque to humans. There is no way to determine (a) how the AI arrived at a decision or prediction, (b) what information is outcome determinative to the AI, or (c) to obtain a ranking of the variables processed by the AI in the order of their importance. Importantly, this form of black box cannot even be analyzed ex post by reverse engineering the AI’s outputs.
Weak Black Boxes: The decision-making process of a weak black box are also opaque to humans. However, unlike the strong black box, weak black boxes can be reverse engineered or probed to determine a loose ranking of the importance of the variables the AI takes into account. This in turn may allow a limited and imprecise ability to predict how the model will make its decisions.
Black box AI models arrive at conclusions or decisions without providing any explanations as to how they were reached. In black box models, deep networks of artificial neurons disperse data and decision-making across tens of thousands of neurons, result in a complexity that may be just as difficult to understand as that of the human brain. In short, the internal mechanisms and contributing factors of block box AI remain unknown.3 Explainable AI, which is created in a way that a typical person can understand its logic and decision-making process, is the antithesis of black box AI.3
One possible reason AI may be a black box to humans is that it relies on machine-learning algorithms that internalize data in ways that are not easily audited or understood by humans. This section provides two illustrative examples. First, a lack of transparency may arise from the complexity of the algorithm’s structure, such as with a deep neural network, which consists of thousands of artificial neurons working together in a diffuse way to solve a problem. This reason for AI being a black box is referred to as “complexity.” Second, the lack of transparency may arise because the AI is using a machine-learning algorithm that relies on geometric relationships that humans cannot visualize, such as with support vector machines. This reason for AI being a black box is referred to as “dimensionality.”
Regulatory and IP Concerns
Regulatory concerns5
From a regulatory standpoint, the AI black box problem presents unique challenges. For starters, the opacity of AI processes can make it increasingly difficult for regulators to assess the compliance of these systems with existing rules and guidelines. Further, a lack of transparency seamingly complicates the ability of regulators to develop new frameworks that can address the risks and challenges posed by AI applications. Lawmakers may struggle to evaluate AI systems’ fairness, bias and data privacy practices, and their potential impact on consumer rights and market stability. Additionally, without a clear understanding of the decision-making processes of AI-driven systems, regulators may face difficulties in identifying potential vulnerabilities and ensuring that appropriate safeguards are in place to mitigate risks.
If we look at Europe, the AI Act aims to create a trustworthy and responsible environment for AI development within the EU. Lawmakers have adopted a classification system that categorizes different types of AI by risk: unacceptable, high, limited and minimal. This framework is designed to address various concerns related to the AI black box problem, including issues around transparency and accountability. The battle is ongoing as Italy banned AI chatbot ChatGPT was for 29 day due to privacy concerns, until an assurance for regulatory compliance was provided by the CEO of the Company.
Addressing the black box problem5
To address the AI black box problem effectively, employing a combination of approaches that promote transparency, interpretability and accountability is essential. Two such complementary strategies are explainable AI (XAI) and open-source models.
Methods often employed in XAI include surrogate models, feature importance analysis, sensitivity analysis, and local interpretable model-agnostic explanations. Implementing XAI across industries can help stakeholders better understand AI-driven processes, enhancing trust in the technology and facilitating compliance with regulatory requirements.
In tandem with XAI, promoting the adoption of open-source AI models can be an effective strategy to address the black box problem. Open-source models grant full access to the algorithms and data that drive AI systems, enabling users and developers to scrutinize and understand the underlying processes.
- ttps://umdearborn.edu/news/ais-mysterious-black-box-problem-explained.
- https://thenextweb.com/news/self-driving-cars-crash-responsible-courts-black-box
- https://www.techtarget.com/whatis/definition/black-box-AI#:~:text=Black%20box%20AI%20is%20any,to%20how%20they%20were%20reached.
- The Artificial Intelligence Black Box and the Failure of Intent and Causation by Yavar Bathaee, Harvard Journal of Law & Technology Volume 31, Number 2 Spring 2018
- https://cointelegraph.com/news/ai-s-black-box-problem-challenges-and-solutions-for-a-transparent-future